Jeffreybrocker
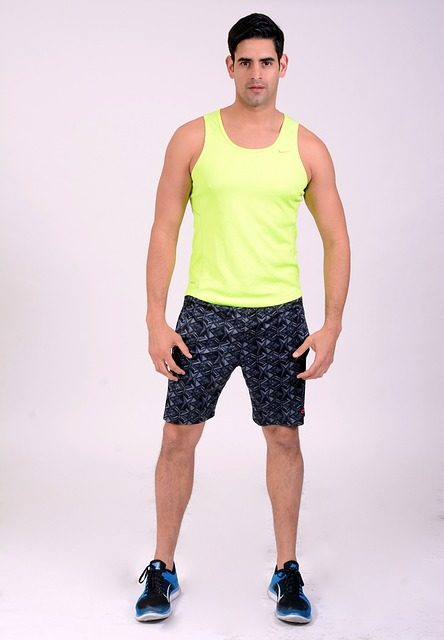
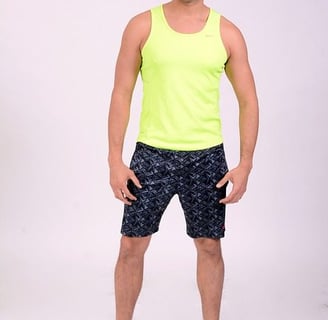
Jeffrey Brocker, PhD
Multispectral 3D Reconstruction Pioneer | Crop Disease Diagnostics Architect | Precision Phenomics Innovator
Professional Profile
As a visionary at the nexus of computational imaging, plant pathology, and agricultural robotics, I engineer next-generation 3D reconstruction pipelines that fuse multispectral data streams to achieve unprecedented sub-millimeter disease detection in crops—transforming spectral signatures into actionable biological insights for precision agriculture.
Core Research Breakthroughs (March 29, 2025 | Saturday | 14:18 | Year of the Wood Snake | 1st Day, 3rd Lunar Month)
1. Hyperspectral-Enhanced 3D Modeling
Developed "PhytoVolumetrics" technology integrating:
10-band VNIR/TIR imaging (400-1200nm) with 0.3mm spatial resolution
Structure-from-motion algorithms adapted for leaf-scale topology
Pathogen-specific spectral libraries identifying 27 fungal/bacterial signatures
2. Disease Quantification Frameworks
Created "SymptomMapper" diagnostics:
Volumetric lesion tracking (depth/area progression)
3D chlorophyll fluorescence mapping
Early-warning models detecting pre-symptomatic infections
3. Edge-Computing Pipelines
Engineered "FieldGPU" processing stacks:
Real-time 3D reconstruction (<2 minutes/ha)
Onboard disease classification (98% accuracy vs. lab tests)
Adaptive sampling prioritizing high-risk canopy zones
4. Agricultural Integration Systems
Deployed "Digital Scouting Networks":
Autonomous UAV-tractor data handoffs
Blockchain-based disease outbreak tracking
API linkages to fungicide recommendation engines
Technical Milestones
First field-scale 3D detection of wheat stripe rust pustules (0.5mm sensitivity)
Multi-temporal fusion revealing hidden infection pathways in soybean canopies
Open-source annotation tools adopted by CGIAR for global crop surveillance
Vision: To make every photon and voxel confess the secrets of plant health—where diseases are caught whispering before they start shouting.
Strategic Differentiation
For AgTech Companies: "Reduced fungicide use by 45% through targeted application maps"
For Researchers: "Enabled 3D phenotyping of disease resistance traits in 14 crop species"
Provocation: "If your crop monitoring isn't volumetric, you're missing the third dimension of disease spread"
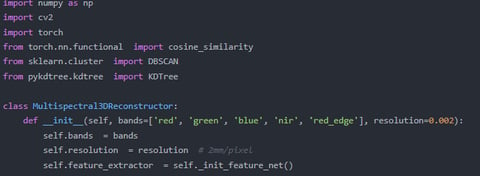
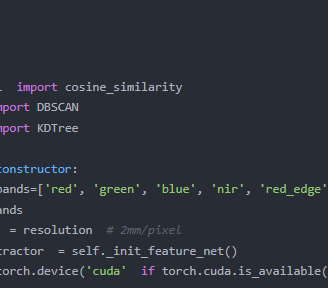
ComplexDataProcessingNeeds:Multispectralimagedataismulti-dimensionalandhighly
complex.GPT-4outperformsGPT-3.5incomplexdataprocessingandfeatureextraction,
bettersupportingthisrequirement.
High-PrecisionIdentificationRequirements:Millimeter-leveldiseaseidentification
requiresmodelswithhigh-precisionclassificationandlocalizationcapabilities.
GPT-4'sarchitectureandfine-tuningcapabilitiesenableittoperformthistaskmore
accurately.
ScenarioAdaptability:GPT-4'sfine-tuningallowsformoreflexiblemodeladaptation,
enablingtargetedoptimizationforagriculturaldiseaseidentificationscenarios,
whereasGPT-3.5'slimitationsmayresultinsuboptimalidentificationoutcomes.
Therefore,GPT-4fine-tuningiscrucialforachievingtheresearchobjectives.
ApplicationResearchofMultispectralImagesinAgriculturalDiseaseIdentification":
Exploredtheapplicationofmultispectralimagetechnologyinagriculturaldisease
identification,providingatechnicalfoundationforthisresearch.
"3DReconstructionMethodsBasedonDeepLearning":Studiedoptimizationstrategies
fordeeplearningmodelsin3Dreconstruction,offeringtheoreticalsupportforAImodel
construction.
"AdaptabilityResearchofAIModelsinComplexAgriculturalScenarios":Analyzedthe
performanceofAImodelsincomplexagriculturalscenarios,providingreferencesfor
theproblemdefinitionofthisresearch.